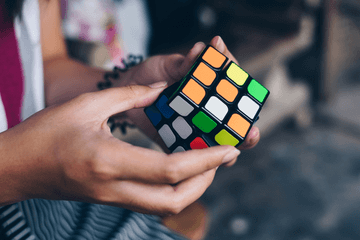
For years, “actionable insights” have been the Holy Grail for data analytics companies. Actionable insights, the thinking goes, are the end product of data collection, aggregation, analysis, and judgment. They enable a decision-maker to modify behavior and achieve desired outcomes.
The process begins with data collection, which can take many forms. There’s a big difference between collecting data and aggregating it in a meaningful way that can provide a picture of reality. That’s the “insights” part of the puzzle. First, you need high-quality data, then you need the technological prowess to clean and organize it.
With high-quality data that’s been cleaned and organized, the next step is to provide context. This is the realm of companies like Tableau, which provide tools that translate machine-friendly data points into human-friendly visualizations that strive to depict an objective picture of current conditions.
But whereas a snapshot of current conditions may, in fact, yield new and meaningful insights (for example, if I look ‘sales numbers’ across an organization I can see which channels are over- or under-performing), human judgment has always been paramount in choosing a particular action. A perfect picture of static conditions doesn’t by itself offer any suggestions as to how to achieve particular outcomes. We still rely on management to tweak sales incentives or redistribute resources.
Or at least we did, up until recently. Machine learning is now shifting the balance of institutional decision-making. Advances in processing and algorithmic self-improvement mean that computers can now anticipate future outcomes and take steps to maximize particular ones. Intelligent systems can now see the world in shades of gray and evaluate likelihoods from multitudes of variables far beyond human comprehension.
That’s the world we currently live in, and the evidence is all around us. Machine learning algorithms have swayed elections by stoking targeted outrage.